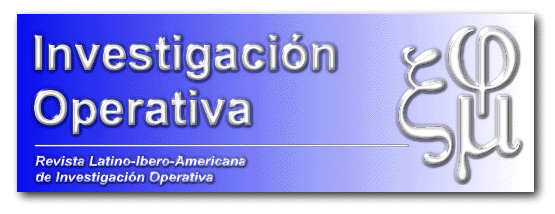
Abstract:
Augmented Lagrangian Methods and Proximal Point Methods for Convex Optimization
We present a review of the classical proximal point method for
finding zeroes of maximal monotone operators,
and its application to augmented Lagrangian methods,
including a rather complete convergence analysis. Next we discuss
the generalized proximal point methods, either with Bregman
distances or $\phi$-divergences, which in turn give raise
to a family of generalized augmented Lagrangians, as smooth
in the primal variables as the data functions are.
We give a
sketch of the convergence analysis for the case of the proximal
point method with Bregman distances for variational inequality problems.
The difficulty with these generalized augmented Lagrangians lies
in establishing optimality of the cluster points of the primal sequence,
which is rather immediate in the classical case. In connection with this issue
we present two results. First we prove optimality of such cluster
points under a strict complementarity assumption (basically that no tight
constraint is redundant at any solution). In the absence
of this assumption, we establish an ergodic convergence
result, namely optimality of the cluster points of a sequence of weighted
averages of the primal sequence given by the method, improving over weaker
ergodic results previously known. Finally we discuss similar ergodic results
for the augmented Lagrangian method with $\phi$-divergences and give the explicit
formulae of generalized augmented Lagrangian methods for different
choices of the Bregman distances and the $\phi$-divergences.